Setup
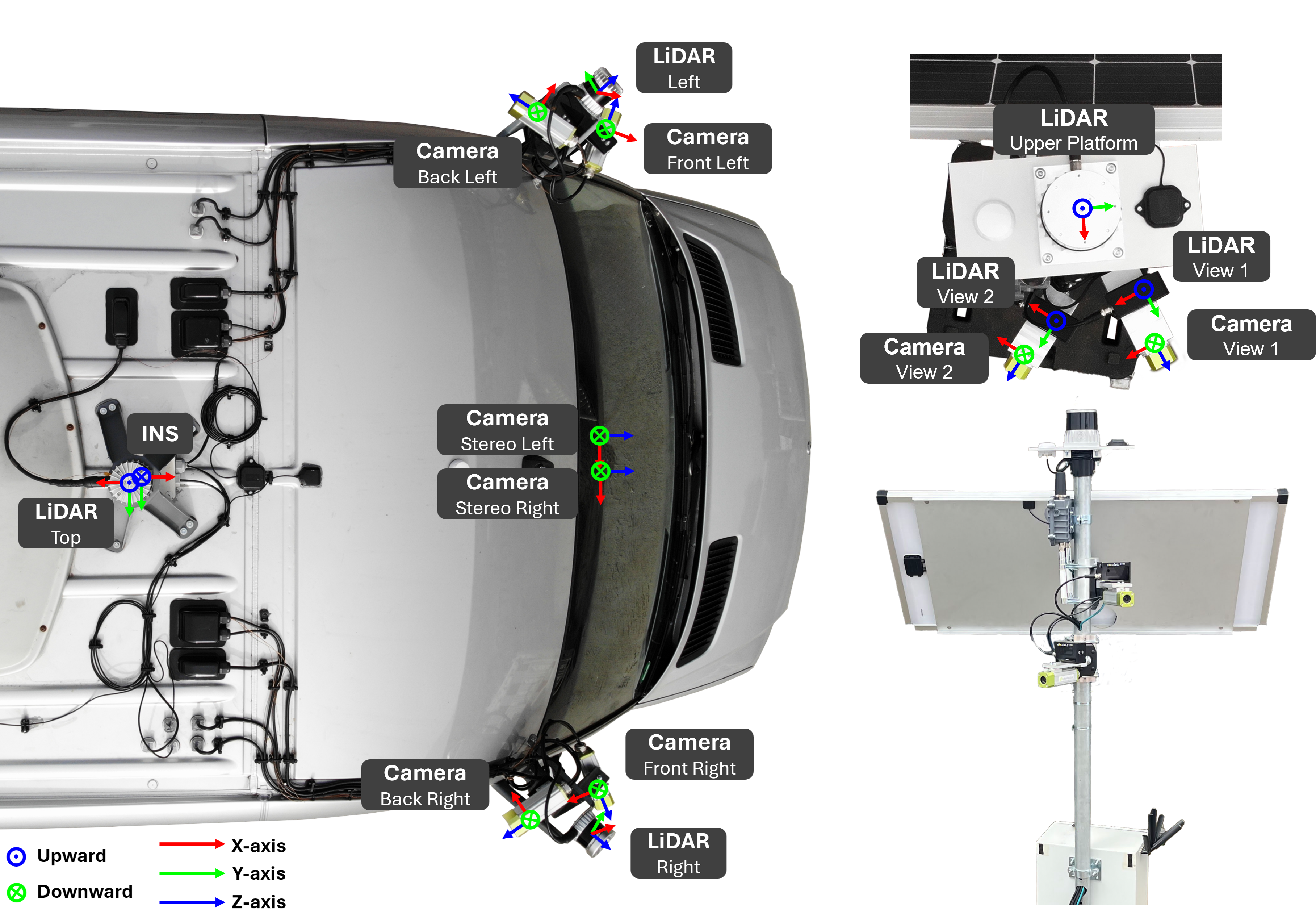
The increasing complexity of urban environments has underscored the potential of effective collective perception systems. To address these challenges, we present the CoopScenes dataset, a large-scale, multi-scene dataset that provides synchronized sensor data from both the ego-vehicle and the supporting infrastructure. The dataset provides 104 minutes of spatially and temporally synchronized data at 10 Hz, resulting in 62,000 frames. It achieves competitive synchronization with a mean deviation of only 2.3 ms. It includes a novel procedure for precise registration of point cloud data from the ego-vehicle and infrastructure sensors, automated annotation pipelines, and an open-source anonymization pipeline for faces and license plates. Covering 9 diverse scenes with 100 maneuvers, the dataset features scenarios such as public transport hubs, city construction sites, and high-speed rural roads across three cities in the Stuttgart region, Germany. The full dataset amounts to 527 GB of data and is provided in the .4mse format and is easily accessible through our comprehensive development kit. By providing precise, large-scale data, CoopScenes facilitates research in collective perception, real-time sensor registration, and cooperative intelligent systems for urban mobility, including machine learning-based approaches.
Our vehicle agent is a modified Mercedes Sprinter, designed with spacious, bus-like seating and an elevated roof for easy entry and comfortable standing room inside the cabin. Equipped with a complete AD perception sensor suite, it features six cameras arranged for 360° coverage, including a secondary front-facing camera optimized for stereo applications (note: there is no dedicated rear camera). The vehicle is also outfitted with three LiDAR sensors to capture mid- and near-range perspectives and a high-precision INS system (integrating GNSS and IMU with correction data) to ensure accurate localization and navigation data.
Our sensor tower shares the same advanced specification as the vehicle agent, serving as a highly adaptable observation unit. It features two movable arms, each mounted with a camera and a solid-state LiDAR unit, alongside a 360° Ouster OS LiDAR (128 rays) positioned at the top for comprehensive coverage. The tower is equipped with a GNSS system and achieves nanosecond-level synchronization with the vehicle’s data stream via PTP, using GNSS-triggered timing. This setup ensures precise alignment of all data within a few milliseconds. Operating independently, the tower relies on a 5G mobile connection, dual 100 Ah (24V) batteries, and a solar panel, making it fully self-sufficient in the field.
For our initial publication we've gathered data from 9 different locations across Stuttgart, Esslingen, and Waiblingen in Baden-Württemberg, Germany. Each location is precisely mapped in a Google Earth project, making it easy to revisit and verify positioning.
Given the varying camera placements and angles on our infrastructure towers, each site requires tailored calibration. At each location, a series of defined maneuvers was driven multiple times to ensure consistency. In total, we offer around 104 minutes of fully synchronized and anonymized data, with our anonymization process and a comprehensive development report to be made publicly available.
We offer 3D RGB point clouds generated by integrating data from LiDAR and cameras, providing detailed spatial and color information for enhanced scene understanding.
A key component of the dataset is the viewpoint from the observing infrastructure, providing a comprehensive perspective from the environment. Every color indicates another sensor.
We provide a high-quality stereo camera system designed for precise depth perception and 3D imaging (front-facing camera only), all accessible through our research and development kit.
For our dataset, we are harnessing offline computing power and advancements in foundation model precision to create high-quality, automatic ground truth annotations. By leveraging the ability to move backward and forward through time in frame sequences, we can generate consistent and accurate labels for the 2020s — where we try to minimize the manual effort. Our pipelines and strategies for this automated process will also be made publicly available, providing a transparent look at the tools and methods behind our data.
The core purpose of our dataset is precise spatial registration, enabling synchronized alignment between observing and moving agents from a geometric perspective. An upcoming experimental study on our approach will be published soon, showcasing our methodology and findings in the area of Collaborative Perception.
We are excited to present our dataset, with a key focus on anonymization to protect privacy. However, recent studies have raised questions about whether object detectors trained on anonymized data perform as effectively on real-world data. To address this, we explored new anonymization methods beyond simple blurring. On this website, we will detail the techniques we used and share the code publicly to support further research and reproducibility.
@misc{vosshans2024aeifdatacollectiondataset,
author = {Marcel Vosshans and Alexander Baumann and Matthias Drueppel and Omar Ait-Aider and Ralf Woerner and Youcef Mezouar and Thao Dang and Markus Enzweiler},
title = {The AEIF Data Collection: A Dataset for Infrastructure-Supported Perception Research with Focus on Public Transportation},
url = {https://arxiv.org/abs/2407.08261},
year = {2024},
}